CACHE : Bridging the Gap Between Molecule Discovery and Computational Design
CACHE, which stands for “Critical Assessment of Computational Hit-finding Experiments”, is a benchmarking effort to obtain high-quality experimental feedback on computational hit-finding predictions using artificial intelligence (AI)/machine learning or physics-based methods. This initiative launched in December 2021 with its first hit-finding challenge – molecule discovery for the LRRK2 gene, which is the most commonly mutated gene associated with Parkinson’s disease.
The CACHE Challenge, which is the hit-finding competition of the Target 2035 initiative, focuses on biologically interesting targets that are selected for hit-finding challenges. Participants computationally search for small molecules that bind to those target proteins. Up to 200 compounds per participant in two learning cycles are purchased or synthesized and tested in two orthogonal assays on the targets, allowing an assessment on the performance of the computational approach used. All data, including chemical structures are made publicly available without restrictions.
Both CACHE and Target 2035 are initiatives led by the Structural Genomics Consortium (SGC), which is a network of scientists in industry and academia, supporting open-access drug discovery around the world. The current SGC research hubs are in Canada, Germany, Sweden, the United Kingdom, and the United States.
Target 2035 is an open science global movement consisting of international scientists and researchers, focusing on the creation of chemical and biological tools for all human proteins by year 2035, with the goal of studying human proteins to inform drug discovery.
CACHE casts a wide and diverse net on the type of participants it seeks contributions from. The initiative invites computational “drug hunters” and algorithm/software developers that are seeking unbiased experimental feedback on their methods and predictions. This may include researchers working in a variety of spaces - academia, biotech, large pharmaceutical companies, or computational chemistry software development companies. Additionally, the CACHE Challenges could also be interesting to pharmaceutical companies or investors that are keen to focus their strategy on top performing technologies.
Each competition lasts about 20 months and includes a hit finding round followed by a hit optimization round. The first CACHE Challenge is underway but there is still time to get involved in CACHE’s maiden molecule-finding quest for the WD40 repeat (WDR) domain of LRRK2. Joining the CACHE Challenge is easy – participants fill out an online application to begin the discovery process. Subsequent challenges will be posted every four months on CACHE’s website.
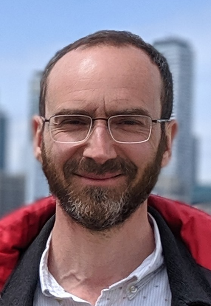
Matthieu Schapira, Principal Investigator at the SGC, University of Toronto and Alexander Hillisch, Vice President, Head of Computational Molecular Design, Wuppertal, Bayer AG Pharmaceuticals, are two researchers who worked on the CACHE research paper. SGC caught up with both researchers to enquire about the CACHE project - its impact on future hit-finding processes and how this initiative will lead to important discoveries for new protein targets, while advancing the open science model of drug discovery. Highlights from the interview are captured below.
Get involved in the first CACHE Challenge. Submit your application by January 31!
Quick CACHE Facts
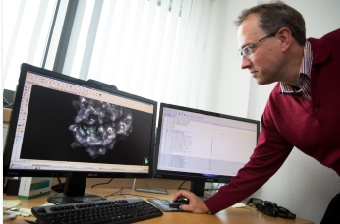
Head of Computational Molecular Design,
Wuppertal, Bayer AG Pharmaceuticals.
What is the overall goal of the project?
AH: The overarching goal of CACHE is to foster computational hit-finding method comparison and development. This is done by providing biologically/pharmacologically useful target challenges and high-quality experimental feedback to small molecule designs. The experimental outcome of CACHE is expected to be also useful for drug discovery in general and initiatives such as Target 2035, aiming at generating a probe molecule for every human protein.
How often are the experiments conducted and awarded?
MS: There will be 3 challenges every year. Everyone is invited to participate. Applications are reviewed by an independent panel via a double-blind process - both participants and reviewers are anonymized.
How is CACHE funded?
MS: Grants. In the future, we hope that disease foundations or pharmacutial companies may be interested in sponsoring competitions focused on particular therapeutic targets where they want to build medicinal chemistry insight in the open. We also believe that future funding pharma members will be interested in advising on the general direction of the initiative. And other groups seeking a window into what is happening in computational drug design may want to join as observers for a fee.
CACHE Science
Where are molecules tested experimentally?
MS: Molecules are tested at the SGC, University of Toronto, where compounds for dozens of targets have been tested in partnership with ten global pharma companies over 15 years.
How are the results shared openly?
MS: At the end of each challenge, which lasts about 20 months, all compound structures, associated data and a generic description of the computational method used to select compounds will be disclosed on cache-challenge.org and also published. The name of participants is anonymized but they will also be encouraged to publish their results separately. We will also organize a symposia twice a year where participants will be invited to present their results.
The first experiment is focused on LRRK2, why did you select this molecule to kick-off the program? What is next?
MS: LRKK2 is the most mutated gene in familial Parkinson’s disease. While current drug candidates target its kinase domain, recent structural data published in Nature suggest that targeting its WDR domain may be a promising alternative. This is the focus of this CACHE challenge. The next challenge will be announced shortly. Targets are selected by an independent target selection committee.
What happens if a pharmaceutical company opts to use the research from one of the CACHE experiments?
AH: CACHE is open science. All data, including chemical structures and molecules screened in silico are made publicly available without restrictions. So, anyone can build on the knowledge that is generated from CACHE.
CACHE Impact
Do you think computational hit-finding initiatives using an open science-based approach will be the way of the future in the drug discovery process?
MS: The holy grail is to invent drugs in silico. I think we will get there eventually, but we are still very, very far. Using open science-based initiatives like CACHE to share scientific insight will collectively get us there faster.
What is the potential impact of CACHE on future research and drug discovery?
MS: If CACHE delivers on its promises, it will define the state-of-the-art as computational hit-finding progresses over the years and will act as an accelerator in the field.
The CACHE Incentive
What are the benefits to researchers coming on-board to conduct these experiments and sharing their results publicly?
MS: Computational drug design experts can access a high-quality platform to test experimentally their predictions, benchmark their methods and potentially demonstrate the superiority of their technology.
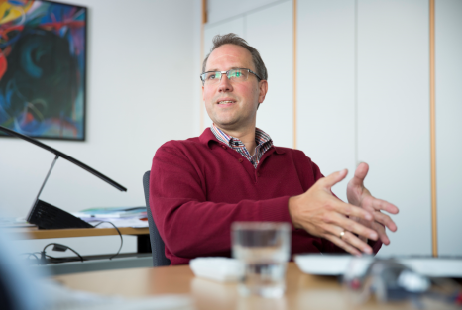
Pharmaceuticals in Wuppertal, Germany.
Why are pharmaceutical companies interested in CACHE?
AH: Pharmaceutical or biotech companies will most likely be interested to get an unbiased, experimentally validated comparison of different computational approaches to hit-finding. This might be the basis for collaborations or the adoption of their own activities in the fields of artificial intelligence, machine learning and physics-based approaches. In addition, information on interesting target proteins might inspire new drug discovery initiatives.
Will pharmaceutical companies seek partnership with top performing groups from CACHE for their internal programs?
AH: This is likely. Consistently performing well on several hit-finding challenges is a good argument for a pharma company to initiate a collaboration or licensing agreement or similar with participants in CACHE. We urgently need more such challenges and robust comparisons to foster data driven decisions when it comes to the artificial intelligence trend.